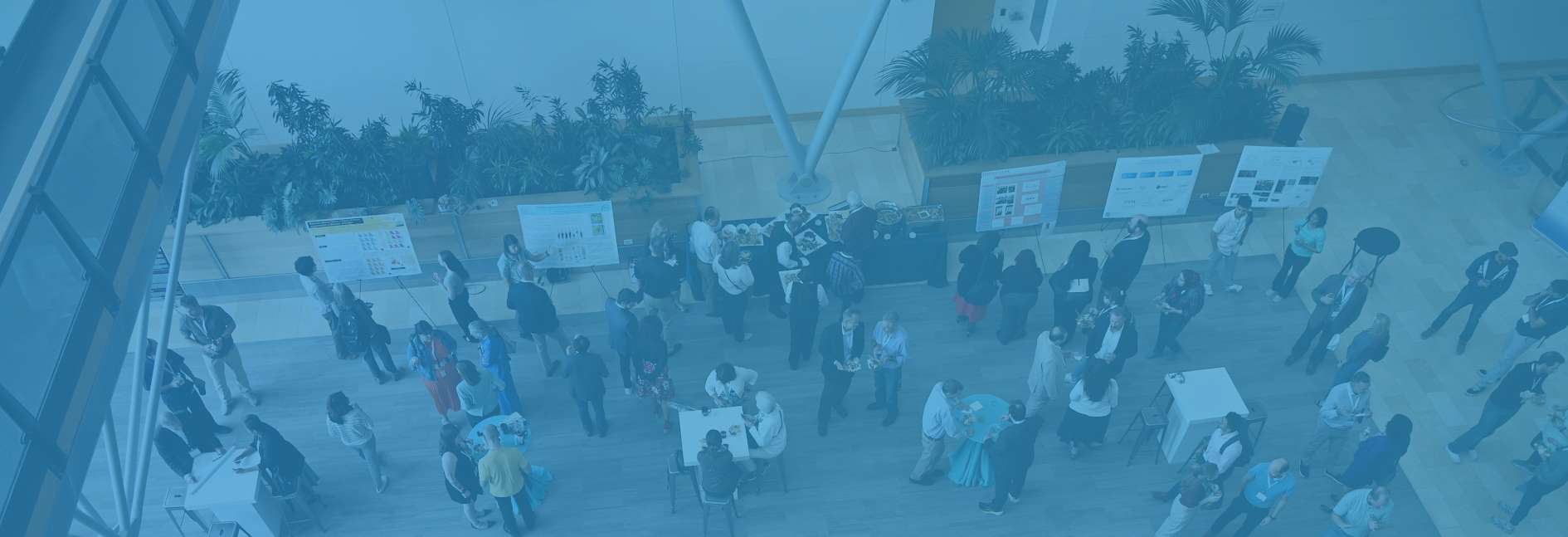
The Geo-Resolution 2024 Student Poster Session
Each year Geo-Resolution hosts a student poster session to help share the new, exciting research that is happening in geospatial across the United States. We welcome posters on a wide range of research topics as long as there is a geospatial aspect to the work. Whether your study uses location science as an analytic mapping tool or your research is breaking new ground in geospatial technologies, we want to highlight your work for the Geo-Resolution audience.
Congratulations to the 2024 Student Poster Competition Winners!
First Place: Emma C. Hall, University of Illinois Urbana-Champaign: “Modeling Vegetation Succession in Response to Thaw Slump Dynamics Using Airborne Hyperspectral Imaging Spectroscopy”
Second Place: Zhijie Zhou, University of Illinois Urbana-Champaign: “CropSight-US from Field(View) to Table: An Object-based Crop Type Ground Truth Dataset Using Street View and Sentinel‑2 Satellite Imagery across the Contiguous United States
Third Place: Ashutosh Pawar, Saint Louis University: “Geospatial Innovations for Industrial Hemp: UAV-based LiDAR and Hyperspectral data fusion for total carbon estimation”
Audience Favorite: Lyric McGilberry, Harris-Stowe State University: “The Darkside of Light: A Study of Contributing Factors to Poor Maternal Fetal Health Outcomes”
2024 List of Student Posters
The Geo-Resolution team is excited to share the following posters from students across the region and beyond. Each poster was selected for its relevance and impact on the geospatial field.
UAV-Based Multitask Learning for Roof Segmentation in Digital Twinning: Urban Planning and Maintenance through Geospatial Analysis
Mohammad Hossein Afsharmovahed, Missouri University of Science & Technology
Poster Abstract: Accurate roof type and material segmentation is essential for urban planning, disaster management, and infrastructure maintenance, especially in rapidly urbanizing regions. Traditional roof inspections are often time-consuming, costly, and unsafe, particularly post-disaster. This study introduces a novel approach that utilizes UAV-based data for simultaneous segmentation of roof types and materials through a multitask learning neural network model. The model employs a shared feature extractor within a multitask framework, with feature decomposition and weight-sharing connections between parallel tasks to enhance accuracy. The backbone architecture is a 32-layer High-Resolution Network (HRNet), pretrained on the PASCAL VOC 2012 dataset. Using HRNet as the shared feature extractor, parallel branches for roof type and material segmentation were designed. A transfer learning technique with a pretrained model was employed, allowing fine-tuning on a smaller pixel-level annotated dataset of UAV images from Missouri S&T campus buildings. The model achieved a mean Intersection over Union (mIoU) of ~85% and accuracy ~95%. The connection defined between the tasks improved accuracy and extracting features once for both branches, reduced the training and inference time. The model’s wight-sharing connection mechanism was validated by demonstrating the logical correlation between the two tasks, supported by consultations with roofing experts, industry standards, and manuals. This research is part of a broader project focused on developing multifunctional models within the digital twin framework for the built environment, underscoring the significant role of geospatial technologies in enhancing urban planning and infrastructure management.
One-Category Transfer Learning on Convolutional Neural Networks for Identifying Fallow Fields from High-Resolution Satellite Imagery
Sk Muhammad Asif, Saint Louis University
Poster Abstract: Fallowing is an agricultural practice that has been leveraged to improve crop production, and soil fertility and support water conservation, especially in the semi-arid or arid regions. Identifying the fallow lands is crucial for food security, provisioning of ecosystem services, and modeling the variations in agricultural water use. Two prominent challenges in identifying fallowing are the wide variety of textures observed during fallowing and limited reporting for fallowing, making its detection challenging compared to other agricultural land use types. This research presents a transfer learning function to identify fallow fields using positive-only fallowing data where the absence of fallowing is unknown. We utilized high-resolution Sentinel‑2 imagery as input and the USDA Cropland Data Layer as ground truth for fallow identification. We have used pretrained models such as Resnet-18, and ResNet-50 which are trained on large Sentinel‑2 datasets. These pretrained models, which contain 13 channels, enable effective transfer learning for identifying fallow fields across diverse agricultural landscapes. Our results show that the proposed transfer function for convolutional neural networks enables task transfer effectively on pre-trained architectures. This approach holds significant potential for large-scale fallow monitoring and could be further refined for application in other regions or integrated with other remote sensing data.
Exploring the relationship between urban green spaces, postpartum depression induced by light pollution and the influence by physical activity.
Samrana Begum, Harris-Stowe State University
Poster Abstract: Research exploring the relationship between green space and postpartum depression exists, yet the mediating role of physical activity remains underexplored. In this study, we investigate the association between PPD and green space exposure, considering the potential mediation effect of physical activity.
Automated Burned Area Delineation from Satellite Imagery
Emma Bennett, University of Missouri-Columbia
Poster Abstract: Wildfires are becoming an increasingly common problem, particularly due to growing effects of climate change. Accurate and automated ways to delineate burned areas are crucial in categorizing the amount of damage and providing context about extent a fire has traveled. Satellite imaging is widespread and readily available, making it an accessible way to obtain fire delineation data. For this experiment, a dataset of Sentinel‑2 satellite images were segmented through application of image processing tools to provide quantification of burned area. A pipeline utilizing the normalized burn ratio, Yen thresholding, local maxima extraction, and a tolerance-based flood fill was developed to accomplish burn area segmentation. Preliminary experiments showed promising results for the tested fires with an average segmentation accuracy of 91%.
Digital-Twin for Disaster Resilience (DTDR)
Jiamin Dang, Missouri University of Science & Technology
Poster Abstract: To achieve equitable and intergenerational resilience under the changing climate, considering the uncertainties in projecting future natural hazards, this study aims to develop robust, evolving digital twins for Disaster Resilience (DTDR) with cutting-edge Geospatial tools and integrated engineering and social science breakthrough discoveries. Among the multi-layer structure of DTDR, the SMART Geospatial Tool is developed to form the building information (BI) database. This tool employs advanced AI and Deep Learning (DL) technologies to accurately extract these critical building attributes (BAs) at a community level by using multi-layer neural networks from Google Street View images (GSVIs), which is critical to predicting the fragility and vulnerability of buildings to natural hazards. The tornadic fragility curve used to predict tornado-induced damage is also enhanced using a realistic tornadic wind field generated by the coupled Cloud Model 1 (CM1) and CFD simulation. This new Geospatial tool will advance Geospatial Science. Implementation of this tool will establish the location-aware datasets for critical BAs needed for resilience planning, enriching the Geospatial database. The developed tool, datasets, and hazard model form the major components of the DTDR for resilience planning, contributing to the shared goal of smart cities and community resilience.
Multidisciplinary Targeting for Remotely Sensed Tailings Dam Deformation
Levi Dial, University of Missouri-Columbia
Poster Abstract: On January 25, 2019, a tailings dam collapsed at Vale S.A.’s Corrego do Feijao iron ore mine in Brumadinho, Brazil. The resulting mudflow traveled over five miles, claiming 270 lives and releasing over 12 million cubic meters of tailings into the surrounding water systems. High concentrations of metal were detected in the water system nearly 200 miles downstream from the original mine site. Disasters of this scale cannot be undone, but they can be prevented. We proposed a multidisciplinary approach to detecting circumstances which could lead to elevated risk of disaster. We aimed to observe public sentiment, financial indicators, and remotely sensed tailings dam deformation leading up to the disaster. Through sentiment analysis of news articles scraped from large industry news outlets, we found that Vale S.A. was spoken about more negatively than positively in the two years preceding the collapse. Using a time-series regression analysis of several financial ratios linked to financial distress, we discovered Vale S.A.’s financial position was steadily degrading leading up to the dam collapse. As a result, Vale S.A. may have been looking to cut costs or hurriedly make use of old operations. In addition to these negative indicators, InSAR from the Alaska Satellite Facility’s Sentinel‑1 imagery showed a hotspot of ground deformation, specifically subsidence, along the tailings dam. This finding alone could represent an early warning sign of instability. This approach could enable remote sensing targeting through more scalable and less computationally expensive methods.
Boundary Bias: How St. Louis’s Geo-Political Boundaries Impact the City’s Violent Crime Rate
Sam Dobson, University of Missouri-St. Louis
Poster Abstract: St. Louis often ranks high in publicized lists like “Most Dangerous Cities in the U.S.” and is known to have a high violent crime rate. However, St. Louis is a unique city in that it is confined to a 66-square mile political boundary (with a population of just over 300,500) and neighbored by the much larger 523-square mile St. Louis County, which has a population of over one million. Taking this into consideration as a researcher, it seems that crime trends in St. Louis are not well-represented with St. Louis City crime data alone, considering that the majority of the population and land area in the St. Louis metropolitan region lies within the County. As a resident of St. Louis County (who lives close to St. Louis City limits), I can personally attest to the confusion and lack of representation this limiting boundary creates regarding crime in St. Louis. I speak for myself, and many of my fellow St. Louisans, when I say that we feel misrepresented as a region by the pictures of crime that are painted by City-only crime rates. This is what has led me to perform my own analysis of violent crime in St. Louis that incorporates crime incident counts from both the City and County, presenting what I feel to be a more accurate representation of the presence of violent crime in our city.
Modeling Vegetation Succession in Response to Thaw Slump Dynamics Using Airborne Hyperspectral Imaging Spectroscopy
Emma C Hall, University of Illinois Urbana-Champaign
Poster Abstract: Arctic landscapes are rapidly changing as permafrost thaws. Retrogressive thaw slumps (RTS) are a type of permafrost mass-wasting feature that alters short and long-term changes in local topography and vegetation cover and abundance. Although broad vegetation succession following RTS disturbance have been explored, the influence of altered microsite topography from RTS activity on patterns of vegetation succession is poorly understood. Here, we use fine-scale remote sensing to map and model the spatiotemporal patterns and trends in plant functional types (PFT) in response to RTS dynamics on the foothills of the Brooks Range, Alaska. We use a combination of aerial and satellite image analysis (1949−2020) and Uncrewed Aerial Systems (UAS) derived hyperspectral and Light Detection and Ranging (LiDAR) data. Random Forest models were used to create 3‑cm spatial resolution PFT maps with overall accuracies ranging 87–92% for each slump. Across all sites, we find the distribution of PFTs to vary with time since disturbance and microtopography. Vegetation mapping finds newly expanded RTS terrain to be characterized by barren soil interspersed with tundra rafts (i.e., vegetation mats collapsed into the RTS scar), mosses and equisetum expand into barren soils after five years, sedges and shrubs establish their dominance after fifteen years, and all early successional PFTs are typically outcompeted by shrubs after >70 years. We link spatial patterns of PFT distribution with topographical gradients within our models to find the distribution of PFTs to be predictable with the relative elevation and RTS slope, likely due to species-specific interactions with tundra raft density. Vegetation succession models may provide a scalable solution using microsite characteristics to predict broad-scale changes in carbon dynamics as RTSs expand across the Arctic.
Developing InSAR Error Models for Geophysical Parameter Retrieval over Permafrost Terrain
Miranda Holloway, Washington University in St. Louis
Poster Abstract: This study is developing error models to improve geophysical parameter retrieval for Arctic permafrost in the Yukon-Kuskokwim delta, along the southwestern coast of Alaska. We utilize interferometric synthetic aperture radar (InSAR) data from the Sentinel-1A satellite to complete this task because of InSAR’s high accuracy and resolution as compared to the distance from the satellite to the target area. In this case, we create interferograms using repeat-pass imaging, comparing the phase of two SAR images to form a single interferogram. With these InSAR datasets, we can visually identify burn scars and other geographical features, and further post-processing continues to clarify the images by removing patchy bodies of water through coherence masking and attaching absolute phase values to the surface movement with phase unwrapping. This semester, we will continue this project by expanding the amount of post-processing applied to the data to further improve statistical clarity and beginning to develop the error models themselves.
Utilizing Open Source Imagery for Humanitarian Mapping
Torin Hovander & UMSL Youth Mappers, University of Missouri-St. Louis
Poster Abstract: UMSL Youth Mappers is a local chapter and the first in the Midwest of a global humanitarian organization that utilizes the power of mapping to empower underserved communities. Our chapter works with chapters from all over the world to address issues ranging from disaster relief to infrastructure development. Our products are utilized by the American Red Cross, British Red Cross, The World Bank, USAID, Bing, Medicins Sans Frontieres, and local governments. Our chapter has been particularly active in flood mapping in Sub-Saharan Africa. This year we are hoping to expand our efforts to flood mapping in Bangladesh. We would like to present our process of collecting, analyzing, organizing, and communicating geographic information. We utilize open-source satellite imagery to designate the locations of important locations. These include but are not limited to, hospitals, roads, bridges, and airports. This information is verified by more experienced analyst. Local Youth Mappers chapters can also verify the information by taking photographs of documented features and geolocating where they took the photo. The data from these maps inform policy makers and relief organizations in developing countries.
Open Source Software at USGS
Kevin Lai, Missouri University of Science & Technology
Poster Abstract: The Hydrography Addressing Tool (HAT) is a critical resource for enabling customers to align their local hydrography data with the National Hydrography Dataset (NHD). Traditionally, our workflow has relied on ArcGIS Online (AGOL) for hosting and editing these datasets. However, as the volume of data increases, AGOL has increasingly become a bottleneck, limiting the efficiency and scalability of our operations. To address this limitation, we explored open-source alternatives to AGOL, specifically focusing on GeoServer and GeoNode. These platforms provide robust and scalable solutions for geospatial data hosting and management without the constraints imposed by AGOL. Through our investigation and implementation, we achieved a significant improvement in data publishing speeds, increasing efficiency by 100 times compared to our previous setup with AGOL. This poster will detail the methodology used to integrate GeoServer and GeoNode into our existing workflow, including data migration strategies, system configuration, and performance benchmarking. We will also discuss the challenges encountered during the transition, such as compatibility issues and the learning curve associated with open-source tools. Our findings suggest that open-source geospatial platforms not only provide a viable alternative to proprietary solutions like AGOL but also offer superior performance in handling large datasets, making them an ideal choice for organizations looking to enhance their geospatial data management capabilities. This poster highlights the potential of open-source solutions in overcoming the limitations of AGOL, providing a roadmap for others facing similar challenges in their geospatial workflows.
The Darkside of Light: A Study of Contributing Factors to Poor Maternal Fetal Health Outcomes
Lyric McGilberry, Harris-Stowe State University
Poster Abstract: This study explores how light pollution impacts maternal health care accessibility, pregnancy success rates, and maternal mental health using Geographic Information Systems (GIS) and Artificial Intelligence (AI). Light pollution, exacerbated by urbanization, negatively affects physical and mental well-being, potentially restricting access to essential maternal health services. The research employs GIS to map health care facilities, population distribution, and light pollution levels, while AI predicts areas with limited access to these services. AI techniques, including predictive modeling, Natural Language Processing (NLP), and image analysis, analyze large datasets to assess how factors like proximity to care, socio-economic conditions, and light pollution correlate with maternal health outcomes. The findings reveal significant disparities in maternal health care accessibility, particularly in urban areas with high light pollution, where reduced access correlates with lower pregnancy success rates and increased mental health issues. AI also aids in understanding patient experiences and the impact of light pollution on sleep, further influencing maternal well-being. The study suggests that policymakers could use these insights to enhance maternal health care access by optimizing facility locations, improving transportation networks, and implementing light pollution mitigation strategies. GIS and AI thus offer crucial tools for addressing disparities in maternal health care access and improving outcomes for mothers and their children.
Intercropping Study to Facilitate Increased Food Production
Jazzmine L. Meekie, Harris-Stowe State University
Poster Abstract: Food insecurity is projected to increase in the face of climate change, and this is especially true for vulnerable populations living in food deserts. Climate change is predicted to impact food productivity which will have major effects on vulnerable populations in urban and rural areas. Reducing food insecurity requires practices that increase crop yield, be adaptable to weather extremes and reduce the carbon footprint. Intercropping, the practice of growing two different crops together has advantages in terms of growing shade tolerant and intolerant plants. If plants with varying moisture needs are grown together, this has the potential for water conservation resulting in increased food production. This research investigates the use of intercropping as a technique to mitigate the effects of climate change in areas designated as food deserts. When different species are grown together moisture is not equal to all water. Our research question explores (a) the level of moisture availability to individual plants within intercropped plants and (b) the effect of shading on intercropped plants. For the moisture experiment, four different types of plants in a single pot and moisture levels were measured twice a day. As control, the 4 plants were grown separately. Seeds were planted in plug flats and then transplanted after 2 weeks into 1‑gallon pots. For this experiment, the first intercropped group had Sunflower, Mustard green, Lettuce, and Garden Bean. The second intercropped group had Sunflower, Mustard Green, Squash, and Cabbage. The plants were drought-stressed, and moisture measurements were taken.
Measuring Road Network Equity and Resilience for Evacuations and Natural Hazards
Alexander Michels, University of Illinois Urbana-Champaign
Poster Abstract: Recent studies across scientific fields reveal that systemic biases can compromise even seemingly objective data. Such biases, especially in disaster management and evacuation planning, have life-threatening implications. Using an optimization model designed to identify bottlenecks in a road network by calculating local worst-case population-to-lane ratios, we identify areas most prone to bottlenecks during emergency evacuations. Through harnessing advanced geospatial computing capabilities, we scaled our analysis to encompass the entire conterminous U.S. We then compared these measures of evacuation vulnerability against the Centers for Disease Control Social Vulnerability Index. Our findings reveal a statistically significant correlation between evacuation and social vulnerabilities and identify clusters of high evacuation and social vulnerability in the southern United States. This underscores inherent biases in the U.S. road infrastructure and the imperative of integrating equity considerations in transportation and evacuation planning.
Using Machine Learning & Geospatial Methods To Develop An Irrigation System Adaptable To Its Environment
Aubree Mitchell, Harris-Stowe State University
Poster Abstract: Water scarcity has long been a concern due to climate change and increased demand for food from growing populations. Urban agriculture is made up of a diverse collection of community rooftops, and backyard gardens plus small commercial farms which serve a vital resource of food in urban areas especially for those communities living in food deserts. Water conservation efforts can contribute to (1) reduced costs of water usage (2) reduced consumption of freshwater sources to promote sustainability and (3) reduced water diversion efforts to protect habitat and biodiversity. Previous research indicates that compared to other plants, sunflowers are more sensitive to soil moisture conditions and wilt faster. Our hypothesis is that computer vision and machine learning will detect wilting based on real time soil moisture levels and indicate the need for irrigation. We designed the experiment by setting up four identical pots of soil then planting seed directly into the pots. The pots were split into two separate groups, one with automated watering, the other, watered manually. The pots were monitored for how much water they received. The experiment was conducted over 8 weeks and results show that the automated watering system triggered watering more frequently compared to watering when wilting was observed manually. While comparing data mid-way through, it was found that the threshold for the automated system was too high and that it could be lowered while maintaining the current health of the plant. Future research ideas include looking at soil composition and testing several types of soil.
Mapping Invasive Thistle and Mustard Using Multispectral Imagery
Lillian Nix, Lindenwood University
Poster Abstract:Invasive plant species such as Italian thistle, milk thistle, and mustard pose significant ecological threats to the blue oak foothills of California. Accurate mapping and monitoring of these species are essential for effective management and conservation efforts. This study utilizes advanced remote sensing techniques, specifically multispectral imagery captured via a senseFly eBee X fixed-wing UAV equipped with a 5‑band camera, to identify and map the distribution of these invasive species. The research was conducted at River Ridge Ranch in Springville, California, an area prone to wildfires and characterized by rough, hard-to-reach terrain, making traditional fieldwork challenging.
Increasing Habitat Classification Accuracy for Javan Slow Loris with Imagery from Drones versus Satellites
Leslie Paige, Washington University in St. Louis
Poster Abstract: Given that discrepancies in land cover estimates have important ramifications for how we understand and protect endangered species’ habitat, this study investigates the potential of drone-based imagery to improve classifications of a complex mosaic landscape that is home to the Critically Endangered Javan slow loris (Nycticebus javanicus). In July 2023, we used a Phantom 4 drone to survey nine loris home ranges (total 118 ha) in Cipaganti, West Java, Indonesia, with a resolution of 2.28 — 4.31 cm/pixel. Drone imagery was classified into land-use land-cover types using object-based image analysis and compared to classifications from high-resolution (3 m) PlanetLabs satellite imagery from the same period. With satellite imagery, forest and labu (Sechium edule), a local crop, were overestimated in most ranges, whereas the total non-labu agricultural area was underestimated. Differences in land-use land-cover estimates between the imagery were statistically significant (Χ2 (3, N = 18) = 2559.8, p < .005) and affected all classification categories (forest, labu, other agriculture, and developed) (Fisher’s exact tests, all p < .005). These differences are relevant for slow loris conservation, as this strictly arboreal species can traverse labu but no other agricultural crops. In addition, satellite imagery could only classify land into general agriculture areas, while drone imagery could distinguish the different agricultural crop types. Although satellite imagery will continue to be a valuable tool for landscape classification, with some species drone-based imagery provides a more accurate characterization of the landscape, which better informs conservation priorities and landscape management decisions.
Geospatial Innovations for Industrial Hemp: UAV-based LiDAR and Hyperspectral data fusion for total carbon estimation
Ashutosh Pawar, Saint Louis University
Poster Abstract: Industrial hemp (Cannabis sativa L.) offers substantial potential for both carbon sequestration and sustainable agriculture, due to its rapid growth, high biomass yield, and significant carbon storage capacity. Additionally, its economic value in generating fibers, seeds, and oil for various industrial applications further increases its appeal. Estimating total plant carbon (TPC), particularly the below-ground biomass (BGB) component, is traditionally challenging due to the complexity of accurately capturing root biomass. This study presents a methodology for estimating TPC, encompassing both above-ground biomass (AGB) and BGB in industrial hemp, by integrating data from Unmanned Aerial Vehicle (UAV)-based Light Detection and Ranging (LiDAR) and hyperspectral remote sensing. Field trials were conducted at the George Washington Carver Research Farm, Lincoln University, Missouri, USA during the summer of 2023. LiDAR-derived vegetation structural metrics, indicative of canopy complexity, and hyperspectral imagery, reflective of plant biochemistry, were collected. In situ measurements of AGB and BGB from destructive sampling were used as ground validation data. These datasets were then used to develop models that estimate TPC. Regression analysis employing various machine learning models was utilized to estimate AGB and BGB. The Support Vector Machine model achieved an R² of 0.87 and an RMSE of 64.52 g/m² when estimating AGB. For BGB estimation, the Random Forest model exhibited the highest accuracy, with an R² of 0.85 and an RMSE of 15.61 g/m². These findings underscore the significant advancement of using remote sensing, coupled with the strong relationship between both AGB and BGB, in estimating total plant carbon. This approach offers a more comprehensive and accurate assessment compared to previous methods that often overlooked the critical contribution of below-ground biomass.
Spatial Analysis of Violent Crime around NGA Locations
Thomas Sandoval, Saint Louis University
Poster Abstract: This study is a spatial analysis of violent crime that occurred from 2021–2023 within 1500 meters of the National Geospatial-Intelligence Agency’s current west campus (NGA) located in the City of Saint Louis and the next campus (N2W) located in North Saint Louis. Using hexagonal grids, the patterns, frequency, and locations were analyzed, compared, and contrasted for the current and future locations of NGA’s campuses.
Deep Learning-Based Segmentation of Buildings and Roads Networks in Complex Urban Scenes
Sara Shojaei, University of Missouri-Columbia
Poster Abstract: Segmentation of buildings and roads plays an important role in many remote sensing applications from mapping, geo-localization, to disaster management. Unfortunately, this is a challenging task due to the complexities of the building and road shapes, distractors in the scene such as vegetation, vary high variance in appearance due to seasons or weather etc. In this work, we are proposing an attention-based novel deep learning network for robust segmentation of buildings and roads in satellite images. Our method focuses on groups of neighboring buildings and the relations between these buildings rather than individual buildings. In parallel, we address the challenges of accurate road segmentation to reduce the errors in automated road network extraction. By addressing both building and road segmentation, our segmentation, our approach significantly improves building pattern recognition and road network extraction. Evaluation on benchmark remote sensing datasets shows that our method outperforms traditional approaches, offering a more robust solution for various applications from mapping to localization in challenging environments.
The Dark Side of Light: Geospatial Analysis and Anatomical Investigation of the Effects of Light Pollution on the Maternal and Fetal outcomes during and after Gestation
Inaya Smith, Harris-Stowe State University/Saint Louis University
Poster Abstract: Exposure to light pollution during pregnancy has been associated with poor gestational, maternal, and fetal health outcomes in mammals. The hypothalamic paraventricular nucleus (PVN) plays a critical role in regulating neuroendocrine changes during pregnancy in rodents. Melatonin, a hormone involved in circadian rhythm regulation, is produced by the pineal gland in response to darkness and has been shown to play an important role in fetal development and maternal health during pregnancy. In this study, we used Geographic Information System (GIS) analysis to identify areas with high levels of light pollution and map them against data on adverse pregnancy outcomes, such as low birth weight and preterm birth. We found that there is a clear relationship between exposure to light pollution and poor pregnancy outcomes. To further our investigation, we looked at data sets centering on different minority status such as educational opportunities, healthcare services and socioeconomic status. These results show a crisis within the Chicago metropolitan area in relation to the availability of proper maternal and fetal care for under served communities. These results will provide important insights into the potential effects of light pollution on maternal and fetal health outcomes, highlighting the need for further research in this area. Moreover, the study further supports the critical role of the PVN in the regulation of pregnancy-related neuroendocrine changes, and the influence of melatonin production on the PVN function during pregnancy. Understanding the molecular mechanisms underlying these effects may have important implications for mammalian maternal and fetal health.
A Novel Tool to Identify Potential Tree Planting Sites and Quantify the Ecosystem Services Provided to Urban Communities and Wildlife
Alyssa Smolensky, University of Missouri-Columbia
Poster Abstract: Rapid urban development combined with the acceleration of climate change presents a significant threat to the welfare of humanity and the natural environment on a global scale. Nature-based solutions, such as urban afforestation, have shown promise as multi-faceted strategies for promoting community resilience and enhancing ecological integrity. However, large-scale urban afforestation projects often struggle to equitably increase tree canopy cover and lack a concrete method for estimating the true impacts of such efforts. This study introduces a dynamic, open-source tool designed to identify optimal tree planting sites within urban environments based on specific goals or desired outcomes. The embedded tree-planting algorithm uses spatial data to search, identify, and evaluate viable planting sites for any given study area. The identified sites are then used to simulate multiple afforestation scenarios to determine which approach is most suitable for achieving the project’s goals. The impacts of each afforestation scenario are quantified and compared by estimating the ecosystem services produced by each planting strategy. Additionally, the effects on local wildlife populations are calculated by modeling changes in landscape connectivity and habitat quality.
CropSight-US from Field(View) to Table: An Object-based Crop Type Ground Truth Dataset Using Street View and Sentinel‑2 Satellite Imagery across the Contiguous United States
Zhijie Zhou, University of Illinois Urbana-Champaign
Poster Abstract: Crop type maps across broad extents are crucial for informing, assessing and managing farming practices in agriculture and food security. Producing such crop type maps usually involves the collection of ground truth data of various crop species, which can be challenging at large scales. Street view images featuring roadside crop fields offer an extensive resource for large-scale crop type ground truth data collection. Here we present the CropSight dataset of object-based crop type ground truth incorporating google street view and Sentinel‑2 imagery covering the contiguous US (CONUS) from 2013 to 2023. The dataset is prepared through a novel deep learning-based modelling framework with three key components: operationalized GSV image collection, UncertainFusionNet model, and Segment Anything Model (SAM). The operationalized GSV image collection systematically acquires representative geotagged GSV images of various crop types spatially sampled at the Agricultural Statistics Districts (ASD) level considering irrigation status (irrigated, rainfed, etc.) and spatiotemporal availability. The UncertainFusionNet model is designed to identify crop types from Google Street View images while also estimating the level of uncertainty in its predictions. The SAM is then fine-tuned and used to delineate the boundaries of associated farm fields with Sentinel‑2 satellite images. Our framework demonstrated overall accuracy above 98.5 % in crop type labelling and over 92.1% (F‑1 score) in crop field boundary delineation across all years and regions. The ground truth dataset identifies 17 representative crop types with labelling uncertainties derived from the UncertainFusionNet model as well as their corresponding crop field boundaries delineated from the fine-tuned SAM. CropSight highlights the potential of integrating GSV and satellite imagery to enhance the accuracy of crop type maps essential for effective agricultural policymaking. By advancing operational capabilities for crop type ground truth retrieval at scales, CropSight contributes to global crop mapping efforts aimed at improving food security analyses from local to global scales.